Appen: The Invisible Infrastructure Powering Generative AI
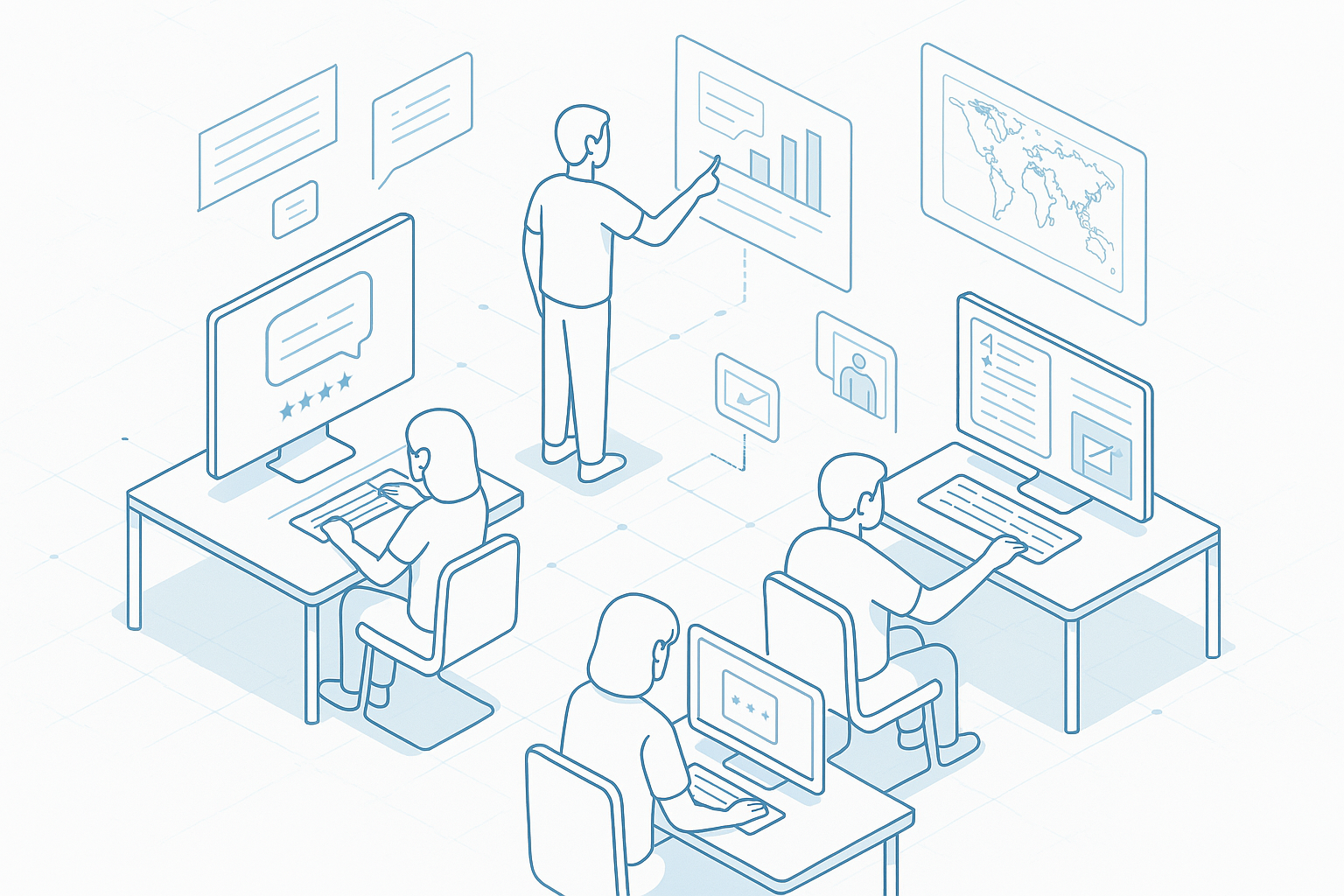
Want to invest in APX?
Visit our How to Invest page to get started with platforms like Fidelity or Robinhood.
Large language models can write, code, and even reason. Computer vision systems can detect tumors and drive cars. Voice assistants can sound human. But behind all of that power — behind the billions of parameters and the breathtaking outputs — is something much less glamorous:
Labeled data. And lots of it.
That’s where Appen (ASX: APX) comes in.
Founded in Australia and now operating globally, Appen is one of the world’s largest providers of human-labeled training data for AI systems. Its workers — sometimes tens of thousands at a time — annotate, classify, tag, and correct the raw material that trains some of the most advanced AI systems on the planet.
If AI is the rocket, Appen is the fuel truck.
The Business: AI Needs Humans, Still
AI models — particularly deep learning systems like GPT, Stable Diffusion, and autonomous driving stacks — need enormous volumes of accurately labeled data. This includes:
● Text with sentiment or intent labels
● Images with object annotations
● Audio with transcription or intent tags
● Videos with action labeling
● Search results with ranking feedback
Appen organizes a global workforce of human annotators to provide this service — helping companies train, test, and refine AI systems across dozens of languages and use cases.
Its core offerings include:
1. Data Collection
Gathering raw data (text, voice, video, sensor) from around the world in different languages, dialects, and contexts.
2. Data Annotation
Tagging and labeling raw data using a mix of human experts and semi-automated pipelines.
3. Model Evaluation
Human-in-the-loop testing of AI models — particularly LLMs — to improve accuracy, safety, and relevance.
4. RLHF (Reinforcement Learning from Human Feedback)
Powering the final tuning stages of LLMs with human reviewers ranking multiple outputs — essential to models like ChatGPT.
Clients and End Markets
Appen’s client list includes:
- Microsoft
- Google / DeepMind
- Meta
- Apple
- Amazon
- NVIDIA
- OpenAI (indirectly via partners)
It also serves:
● Autonomous vehicle companies (e.g., labeling road scenes)
● Voice AI and transcription platforms
● Government agencies working on AI language and translation
● Healthcare startups building annotated radiology and pathology datasets
These clients use Appen for:
● Pre-training data creation
● Fine-tuning
● Bias and toxicity reduction
● Multilingual model expansion
In short: no data, no AI — and Appen is often the first step in the pipeline.
The AI Boom: And the Whiplash
Between 2015 and 2021, Appen was a hypergrowth success story — riding the first wave of deep learning and the platform wars. Revenue soared from under $100M AUD to over $600M AUD. Its shares climbed as major U.S. tech companies leaned into massive model development.
But 2022–2023 brought a correction. As generative AI ramped up, tech companies shifted toward in-house datasets and direct hiring, while automation tools began eating into basic annotation tasks.
Appen responded with:
● Layoffs
● Executive reshuffling
● Platform consolidation
● Increased investment in automated + human hybrid workflows
● More focus on high-quality, high-context work (e.g., RLHF vs. basic annotation)
Now, with new LLMs launching monthly and AI arms races heating up again, Appen is positioning itself as the high-fidelity, human-feedback specialist.
AI Relevance: Human-in-the-Loop at Scale
The more powerful models become, the more refined and ethical their training must be. That’s where Appen adds value.
Key Areas:
● RLHF: Models like ChatGPT, Claude, and Gemini use reinforcement learning from humans to make answers more helpful, polite, and non-toxic
● Multilingual AI: Appen’s global annotator network covers 180+ languages and dialects — vital for expanding LLMs into underrepresented geographies
● Bias & Fairness Auditing: Human reviewers identify stereotypes, hallucinations, and edge cases
● Contextual Ranking: Fine-tuning models to prioritize useful over factual but irrelevant answers
AI doesn’t stop needing people. It just needs better-trained people — and at scale.
Financials: Restructuring Mode, Seeking a Rebound
Appen has had a rocky ride over the past three years — but it’s now refocusing for the next chapter of AI growth.
● Market Cap (Q2 2025): $170M AUD ($110M USD)
● 2024 Revenue: ~$275M AUD (down from peak, but stabilizing)
● Gross Margin: ~30%
● Cash on Hand: ~$30M AUD
● Net Income: Negative (losses due to restructuring and margin pressure)
● Customers: Over 100 active global tech + enterprise AI clients
● Workforce: 1M+ registered contributors globally, with ~100K active at any time
The company has said its goal in 2025 is to return to profitability, reduce project volatility, and increase exposure to RLHF and multimodal AI clients.
Competitive Landscape: Data Is a Crowded Field
Appen’s long-standing advantage — its massive, globally distributed and well-managed annotator workforce — is now being tested by a wave of automation and new entrants. Several emerging players are competing across different segments of the data labeling market:
Scale AI has taken a tech-first approach, positioning itself as a Silicon Valley leader in ML ops and high-velocity data labeling.
Lionbridge AI brings deep strength in language translation and global reach, especially for localization and annotation projects.
Samasource stands out for its ethical sourcing model, employing workers in Africa and emphasizing its social mission.
TELUS International AI bundles its human-in-the-loop services with broader customer experience (CX) offerings, creating an end-to-end service suite.
Amid this competition, Appen remains uniquely strong in several areas:
- Deep language diversity and localization experience
- Enterprise-grade compliance and quality control
- Long-standing relationships with major tech platforms
- Proven ability to scale complex annotation projects across verticals
Appen’s future hinges on its ability to move up the value chain — doubling down on RLHF, personalization, and the increasingly specialized demands of next-generation model training.
Want to invest in APX?
Visit our How to Invest page to get started with platforms like Fidelity or Robinhood.
Disclosure: This article is editorial and not sponsored by any companies mentioned. The views expressed in this article are those of the author and do not necessarily reflect the official policy or position of NeuralCapital.ai.